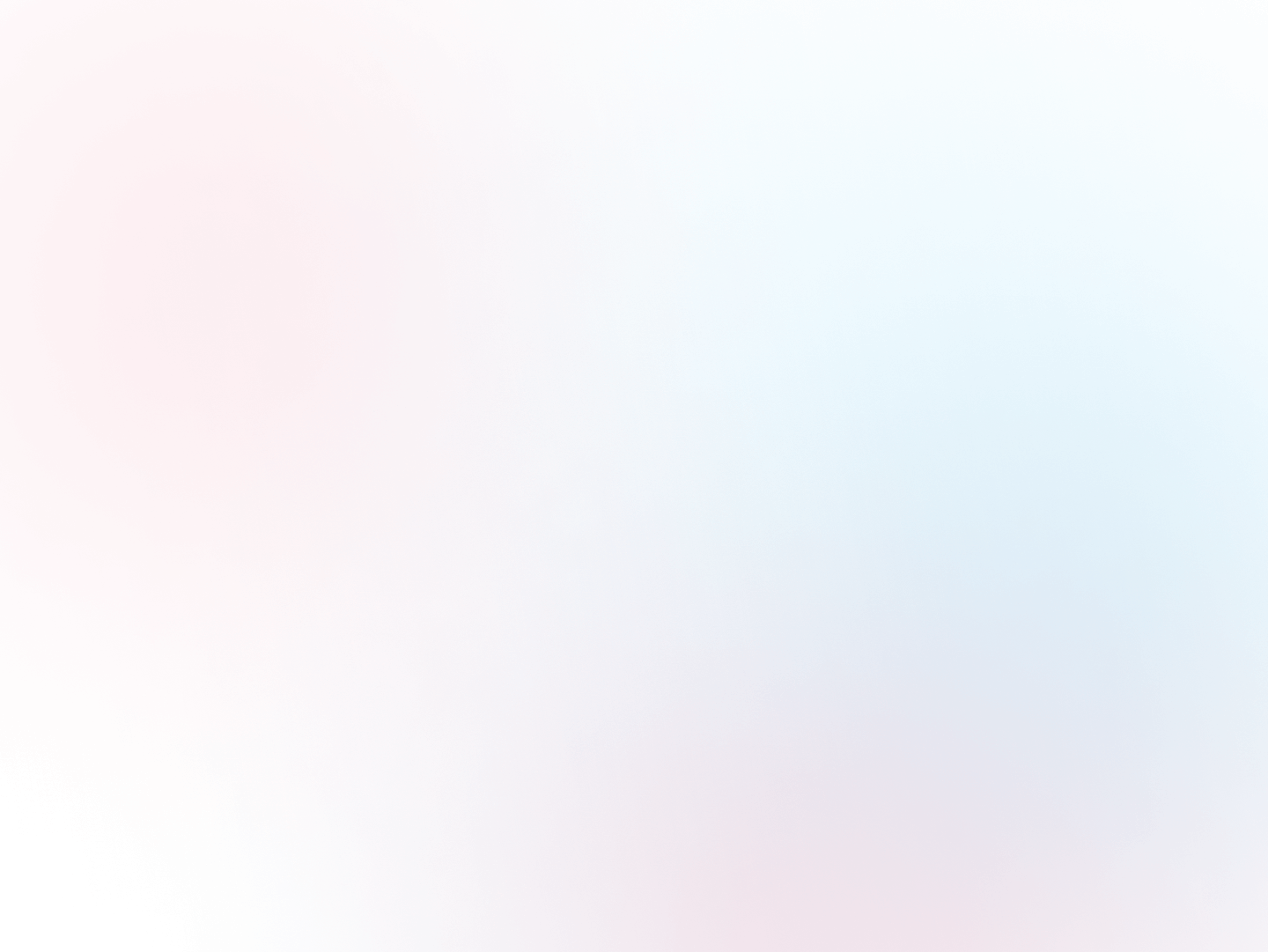
Deep learning text analysis - A valuable tool for feedback
- 30 04 2021
- in
- by Monique van Geest
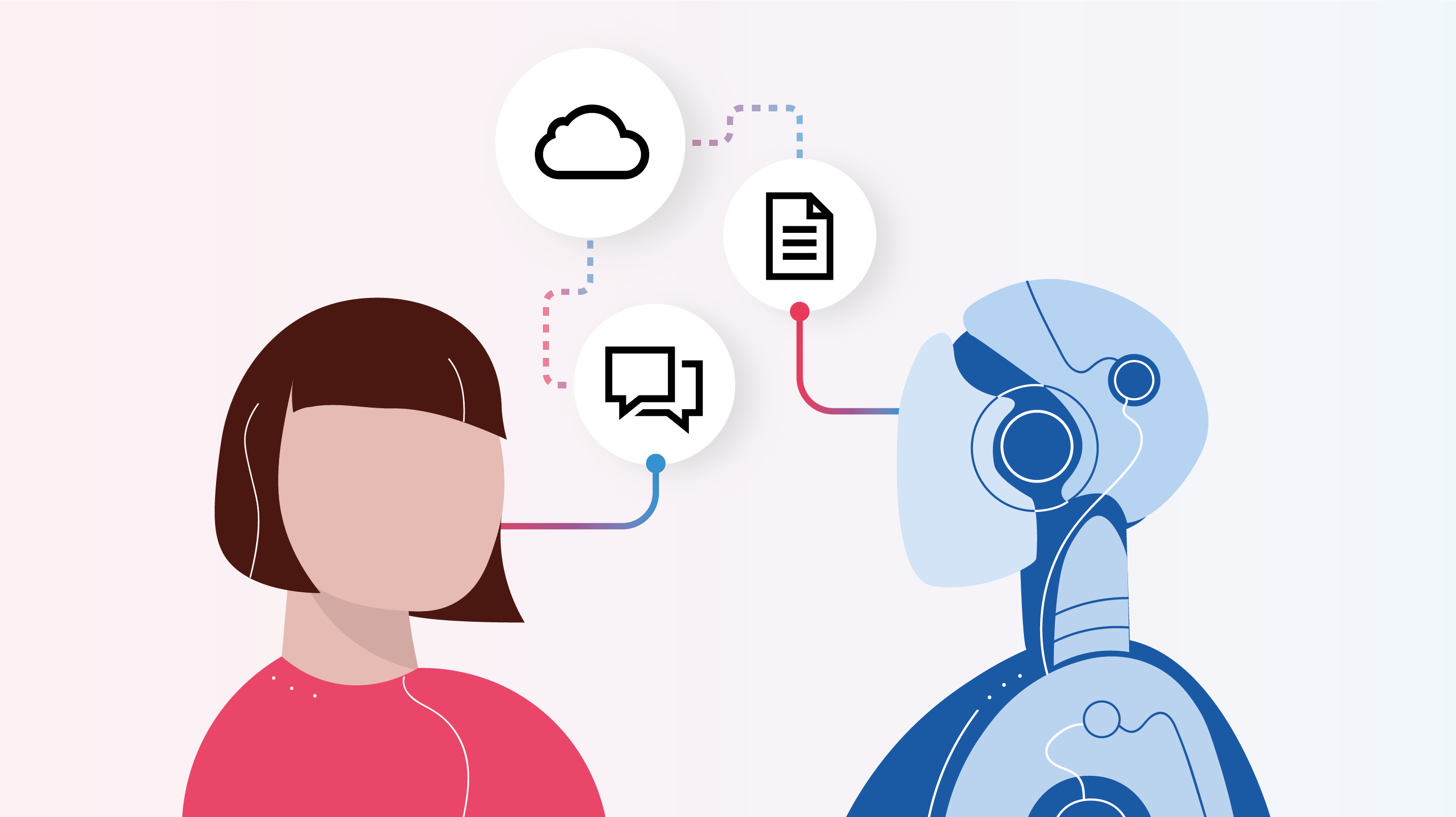
Going through hundreds of texts, looking for the common thread in customer feedback. A time-consuming job that is often put off. Yet it is important. After all, you don't ask for open feedback for nothing. Because where multiple choice questions push the reader into a box, open text allows them to freely express their opinion. This, in turn, enables you to receive reliable feedback and implement targeted improvements.
Text analysis comes in handy when analyzing open feedback. It brings you order out of chaos, saves you time and gives you quick results. Sounds like a golden duo, doesn't it? We will tell you all about it in this article.
Natural Language Processing
Text analysis is part of Natural Language Processing (NLP) and helps organizations categorize open feedback. NLP is a branch of artificial intelligence that helps computers to understand, interpret and manipulate human language. NLP can be used for many different purposes.
There are many applications of NLP in our daily life that you might not immediately think of. Conversations with virtual assistants like Siri on your iPhone for example. Through speech recognition, Siri responds to you when you call his or her name. The virtual assistant is programmed in such a way that certain words and sentences trigger a response! Besides this example, you can find more examples below:
- A voicemail message that you can read typed out in your mailbox or telephone. This is an NLP application called speech-to-text conversion.
- Do you ever navigate a website by using a search bar? Or clicking on a certain tag? For this, we also filter through text using an NLP method. This is called topic modelling.
The above examples are relatively easy to set up. Do you want to go deeper? Then sentiment analysis or machine translation come to the forefront. You will read more about sentiment analysis later.
Order in a jumble of data with text analysis
Nothing is as complex and diverse as language. There are now about 6000 different languages spoken around the world. In addition, there are different dialects, accents and writing styles. Can computers find order in all this? With deep learning text analysis, this is certainly possible. Consider an organization that requests feedback from all over Europe. It is almost impossible to analyze all the feedback with attention. This is where topic detection comes in. First, you determine the groups into which you want to divide your reviews. About which products or subjects is a lot of feedback given? For example, an electronics shop will want to know how satisfied customers are with their new laptop, tablet or smartphone. With the tooling of Insocial this is easily labeled and the reviews are classified by topic.
You can analyze data from e-mails, surveys and service transcripts, for example. It saves you a lot of research, which you can spend on things that a computer cannot handle!
Attention to emotions and opinions with sentiment analysis
When you want to detect emotions and moods, sentiment analysis is important in addition to text analysis. Sentiment analysis examines the subjective information contained in texts. They detect words in which emotions, moods and opinions are expressed about a certain subject or person. Sentiment analysis is also a part of NLP. Again, the computer scans different types of texts looking for patterns and classifying the different types of emotions into groups. Unprocessed text is analyzed for positive and negative expressions. Our models are pre-trained and for selected languages, a review is analyzed and evaluated in no time!
Aspect based analysis
To successfully analyze and assess all open feedback, you will use topic detection and sentiment analysis. This is called "aspect based analysis". Whereas text analysis practically scans texts and sentences and groups them together, with sentiment analysis you look for words that indicate an emotion or opinion. This allows you to categorize your feedback into positive, neutral and negative. In this way, you will be able to recognize trends and see at a glance who is complaining and how many respondents are saying positive things about your brand. So, save time and money and let the computer do the work!
-
-
-
Structure your data easily
-
Recognize negative feedback from different channels on time
-
Find out which feedback occurs frequently
-
Recognize patterns
-
Save time, money & work
-
-
Getting started with text analysis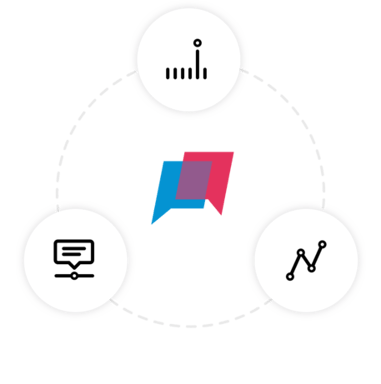
With Insocial you can get started with deep learning text analysis! As practiced feedback tooling, we are experts in analyzing reviews and improving customer experience! Next to text analysis we do a lot more. Wondering what we can do for your organization? Then request a no-obligation demo and talk to an expert.